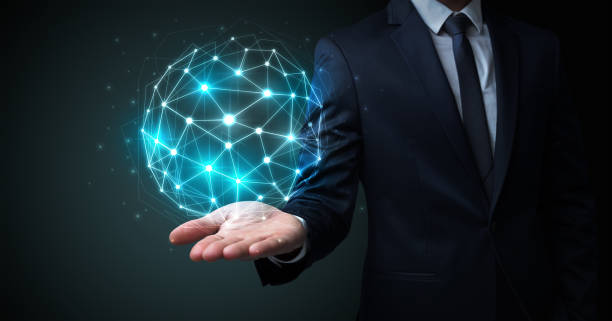
Explainable AI
Artificial Intelligence (AI) has transformed numerous industries, revolutionizing the way tasks are performed and decisions are made. However, one area of concern has been the lack of transparency and interpretability in AI systems. Artificial Intelligence (AI) has transformed numerous industries, revolutionizing the way tasks are performed and decisions are made. In this article, we delve into the world of Explainable AI, understanding its importance, challenges, and the potential it holds for building trust and accountability in AI-driven systems.
The Need for Explainable AI:
As AI systems become increasingly complex, there is a growing demand for transparency and accountability in their decision-making processes. The lack of interpretability in traditional “black box” AI models poses challenges, especially in high-stakes domains such as healthcare, finance, and autonomous vehicles. Stakeholders require insights into how AI models arrive at their decisions to ensure fairness, avoid bias, and maintain ethical standards. Explainable AI bridges this gap by providing understandable explanations for AI-driven outcomes, enabling humans to trust and validate the decisions made by AI systems.
Challenges in Explainable AI
Model Complexity:
Many AI models, such as deep neural networks, are highly complex with millions of parameters, making it difficult to understand how they arrive at specific decisions. Simplifying and explaining these complex models without sacrificing accuracy is a significant challenge.
Trade-off between Accuracy and Explainability:
There is often a trade-off between model accuracy and explainability. Highly interpretable models may sacrifice performance, while more accurate models may be less interpretable. Striking the right balance between the two is a crucial challenge in XAI.
Contextual Understanding:
Providing explanations that are meaningful and relevant to end-users requires an understanding of the context in which the AI system operates. Capturing this context and tailoring explanations accordingly is a non-trivial task.
Approaches to Explainable AI:
Rule-based Explanations:
Rule-based models explicitly define decision rules, making them highly interpretable. They provide explanations in the form of logical rules or decision trees that outline how specific features or inputs influence the final decision.
Local Explanations:
Local explanations focus on explaining the behavior of AI models for specific instances or predictions. Techniques like LIME (Local Interpretable Model-Agnostic Explanations) highlight the most influential features for a particular prediction, providing insights into the decision-making process.
Model-agnostic Approaches:
Model-agnostic methods aim to explain the predictions of any AI model, regardless of its underlying architecture. They focus on understanding the inputs’ impact on the output without relying on detailed knowledge of the model’s internal workings.
Interactive Explanations:
Interactive explanations allow users to explore and interact with AI models to gain a better understanding of their decision-making process. This can involve visualizations, sensitivity analysis, or interactive interfaces that enable users to probe the model and evaluate its behavior.
Benefits of Explainable AI:
Trust and Accountability:
By providing transparent explanations, XAI fosters trust in AI systems. Users can understand the factors influencing decisions and verify the fairness and ethicality of the outcomes.
Detecting Bias and Discrimination:
Explainable AI enables the identification of biases and discriminatory patterns in AI models. By understanding the decision process, it becomes possible to rectify and mitigate these biases, ensuring fairness and inclusivity.
Regulatory Compliance:
In regulated industries, such as healthcare and finance, explainability is often a legal requirement. XAI facilitates compliance with regulations by providing auditable and understandable decision-making processes.
Collaboration between Humans and AI:
Explainable AI allows humans to work alongside AI systems more effectively. Users can comprehend the reasoning behind AI suggestions and make informed decisions based on both human expertise and AI insights.
Also Read: Quantum Computing: Unleashing the Power of the Quantum Realm
Methods and Techniques in Explainable AI:
Feature Importance:
This method focuses on identifying the most influential features or inputs that contribute to the AI model’s decision. Techniques like feature attribution or saliency maps highlight the contribution of each feature to the final outcome.
Rule Extraction:
Rule extraction approaches aim to extract human-understandable rules from complex AI models. These rules provide insights into how specific combinations of features lead to certain decisions.
Model Visualization:
Visualization techniques help users understand the inner workings of AI models by providing visual representations of the model’s structure and decision process. Visualizations can include decision trees, heatmaps, or network graphs that illustrate how information flows through the model.
Prototypes and Counterfactuals:
Prototypes are representative instances that exemplify a particular class or decision, helping users understand the characteristics that define that class. Counterfactuals are alternate instances that demonstrate how changing specific features would affect the model’s decision.
Local and Global Explanations:
Local explanations focus on explaining individual predictions, while global explanations provide insights into the overall behavior of the AI model. Local explanations help users understand why a specific prediction was made, while global explanations provide a broader view of how the model behaves across different instances.
Applications of Explainable AI:
Healthcare:
XAI can support medical professionals in understanding and validating AI-driven diagnoses or treatment recommendations. It can provide explanations for medical predictions, enabling doctors to make informed decisions and improving patient trust.
Finance:
In the financial industry, explainable is crucial for risk assessment, fraud detection, and credit scoring. XAI can provide explanations for credit decisions, investment recommendations, and anomaly detection, allowing stakeholders to understand the factors influencing those decisions.
Autonomous Systems:
In domains such as self-driving cars or drones, XAI is vital for ensuring safety and accountability. By providing transparent explanations for the decisions made by autonomous systems, XAI helps users understand why certain actions were taken and facilitates trust in these technologies.
Legal and Regulatory Compliance:
Explainable is often required to comply with legal and regulatory frameworks. XAI can provide auditable and understandable decision-making processes, ensuring compliance in areas such as healthcare, insurance, and algorithmic fairness.
Customer Service and Personalization:
XAI can help businesses provide personalized recommendations and customer service by explaining why certain products or services were suggested. This enhances customer understanding and satisfaction.
Challenges and Future Directions:
Balancing Accuracy and Explainability:
Striking the right balance between model accuracy and explainability remains a challenge. There is ongoing research to develop methods that are both highly accurate and interpretable.
Human-Computer Interaction:
Designing intuitive and effective interfaces for presenting explanations is crucial to ensure users can easily understand and utilize the provided information.
Ethical Considerations:
The ethical implications of XAI should be carefully addressed. For example, providing explanations to malicious actors may enable them to exploit vulnerabilities in the model.
Domain-Specific Explanations:
Different domains require tailored explanations. Developing XAI techniques that can adapt to specific contexts and user needs is an area of active research.
While Explainable AI offers numerous benefits, it’s important to consider potential risks and drawbacks associated with its implementation.
Here are some of them:
Complexity and Accuracy Trade-Off:
Achieving high accuracy and full explainability can be challenging. In certain cases, highly interpretable models may sacrifice accuracy, while more accurate models may be less interpretable. Striking the right balance between accuracy and explainability is a crucial challenge in XAI.
Limited Interpretability:
Although XAI aims to provide explanations for AI system decisions, the level of interpretability may vary depending on the complexity of the model. Some AI models, such as deep neural networks, may still be difficult to fully understand and explain due to their intricate structure and numerous parameters.
Overreliance on Explanations:
Users of AI systems may become overly reliant on the explanations provided by XAI, assuming they are always accurate and complete. However, explanations can still be subject to biases or limitations, and blindly accepting them without critical evaluation may lead to erroneous conclusions.
User Misinterpretation:
The interpretation of explanations can be subjective and prone to misinterpretation. Users may misjudge or misattribute certain features or factors as the sole cause of a decision, leading to a misunderstanding of the underlying AI system’s behavior.
Increased Complexity and Development Time:
Integrating XAI into AI systems can introduce additional complexity and development time. Building interpretable models or implementing explanation techniques may require specialized expertise and resources, potentially increasing the overall complexity of the AI system.
Security and Privacy Risks:
Exposing the inner workings of AI models through explanations may create security and privacy risks. Adversaries may exploit this information to manipulate or attack the model, potentially leading to compromised system performance or unauthorized access to sensitive data.
Ethical Considerations:
Ethical concerns arise when providing explanations to individuals who may misuse them. Explanations could be used to reverse-engineer proprietary models or enable malicious actors to exploit vulnerabilities, posing risks to intellectual property or system integrity.
Regulatory Compliance Challenges:
Implementing XAI may present challenges in meeting regulatory requirements. Different industries have specific regulations governing transparency, fairness, and accountability, and ensuring compliance with these regulations can be complex.
It’s crucial to address these risks and drawbacks through careful design, ongoing research, and collaboration between experts in AI, ethics, and legal domains. Striking the right balance between transparency, accuracy, usability, and security is essential to effectively harness the benefits of XAI while mitigating potential risks.
Explainable AI is a critical field that aims to provide transparency, accountability, and trust in AI systems. Through various methods and techniques, XAI offers human-understandable explanations for AI-driven decisions, enabling users to comprehend and validate the outcomes. The applications of XAI are vast, ranging from healthcare and finance to autonomous systems and legal compliance. As research and development in the field progress, addressing challenges and ethical considerations will be crucial to harness the full potential of XAI and build a future where AI systems are explainable, transparent, and aligned with human values.
In Conclusion, Explainable AI represents a pivotal step towards building transparent and accountable AI systems. By providing human-understandable explanations, XAI addresses the challenges posed by complex black box models. It empowers users to trust AI systems, detect biases, ensure fairness, and comply with regulations. As the field continues to advance, a greater emphasis on explainability will drive the responsible and ethical deployment of AI, fostering a harmonious collaboration between humans and intelligent machines, and shaping a future where AI decisions are transparent and comprehensible.